A precision brain-controlled prosthesis nearly as good as one-finger typing
August 4, 2015
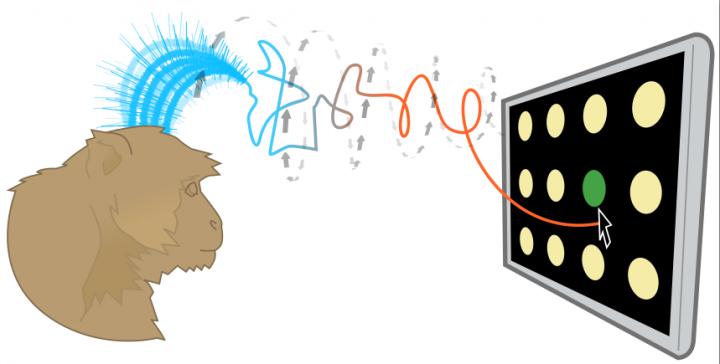
Brain-controlled prostheses sample a few hundred neurons to estimate motor commands that involve millions of neurons. So tiny sampling errors can reduce the precision and speed of thought-controlled keypads. A Stanford technique can analyze this sample and quickly make dozens of corrective adjustments to make thought control more precise. (credit: Jonathan Kao, Shenoy Lab)
An interdisciplinary team led by Stanford electrical engineer Krishna Shenoy has developed a technique to improve brain-controlled prostheses. These brain-computer-interface (BCI) devices, for people with neurological disease or spinal cord injury, deliver thought commands to devices such as virtual keypads, bypassing the damaged area.
The new technique addresses a problem with these brain-controlled prostheses: they currently access a sample of only a few hundred neurons, so tiny errors in the sample — neurons that fire too fast or too slow — reduce the precision and speed of thought-controlled keypads.
Understanding brain dynamics for arm movements
In essence the new prostheses analyze the neuron sample and quickly make dozens of corrective adjustments to the estimate of the brain’s electrical pattern.
Shenoy’s team tested a brain-controlled cursor meant to operate a virtual keyboard. The system is intended for people with paralysis and amyotrophic lateral sclerosis (ALS), also called Lou Gehrig’s disease, a condition that Stephen Hawking has. ALS degrades one’s ability to move.
The new corrective technique is based on a recently discovered understanding of how monkeys naturally perform arm movements. The researchers studied animals that were normal in every way. The monkeys used their arms, hands and fingers to reach for targets presented on a video screen. The researchers sought to learn, through hundreds of experiments, what the electrical patterns from the 100- to 200-neuron sample looked like during a normal reach — to understand the “brain dynamics” underlying reaching arm movements.
“These brain dynamics are analogous to rules that characterize the interactions of the millions of neurons that control motions,” said Jonathan Kao, a doctoral student in electrical engineering and first author of the open-access Nature Communications paper on the research. “They enable us to use a tiny sample more precisely.”
A decoding algorithm
In their current experiments, Shenoy’s team members distilled their understanding of brain dynamics into an algorithm that could decode (analyze) the measured electrical signals that their prosthetic device obtained from the sampled neurons. The algorithm tweaked these measured signals so that the sample’s dynamics were more like the baseline brain dynamics and thus more precise.
To test this algorithm, the Stanford researchers first trained two monkeys to choose targets on a simplified keypad. The keypad consisted of several rows and columns of blank circles. When a light flashed on a given circle the monkeys were trained to reach for that circle with their arms.
To set a performance baseline, the researchers measured how many targets the monkeys could tap with their fingers in 30 seconds. The monkeys averaged 29 correct finger taps in 30 seconds.
In the actual experiment, the researchers only scored virtual taps that came from the monkeys’ brain-controlled cursor. Although the monkey may still have moved his fingers, the researchers only counted a hit when the brain-controlled cursor, corrected by the algorithm, sent the virtual cursor to the target.
The prosthetic scored 26 thought-taps in 30 seconds, about 90 percent as quickly as a monkey’s finger. (See video of hand versus thought-controlled cursor taps.)
Thought-controlled keypads are not unique to Shenoy’s lab. Other brain-controlled prosthetics use different techniques to solve the problem of sampling error. But of several alternative techniques tested by the Stanford team, the closest resulted in 23 targets in 30 seconds.
Next steps
The goal of all this research is to get thought-controlled prosthetics to people with ALS. Today these people may use an eye-tracking system to direct cursors or a “head mouse” that tracks the movement of the head. Both are fatiguing to use. Neither provides the natural and intuitive control of readings taken directly from the brain.
“Brain-controlled prostheses will lead to a substantial improvement in quality of life,” Shenoy said. “The speed and accuracy demonstrated in this prosthesis results from years of basic neuroscience research and from combining these scientific discoveries with the principled design of mathematical control algorithms.”
The U.S. Food and Drug Administration recently gave Shenoy’s team the green light to conduct a pilot clinical trial of their thought-controlled cursor on people with spinal cord injuries.
“This is a fundamentally new approach that can be further refined and optimized to give brain-controlled prostheses greater performance, and therefore greater clinical viability,” Shenoy said.
Abstract of Single-trial dynamics of motor cortex and their applications to brain-machine interfaces
Increasing evidence suggests that neural population responses have their own internal drive, or dynamics, that describe how the neural population evolves through time. An important prediction of neural dynamical models is that previously observed neural activity is informative of noisy yet-to-be-observed activity on single-trials, and may thus have a denoising effect. To investigate this prediction, we built and characterized dynamical models of single-trial motor cortical activity. We find these models capture salient dynamical features of the neural population and are informative of future neural activity on single trials. To assess how neural dynamics may beneficially denoise single-trial neural activity, we incorporate neural dynamics into a brain–machine interface (BMI). In online experiments, we find that a neural dynamical BMI achieves substantially higher performance than its non-dynamical counterpart. These results provide evidence that neural dynamics beneficially inform the temporal evolution of neural activity on single trials and may directly impact the performance of BMIs.