Using animal training techniques to teach robots household chores
May 18, 2016
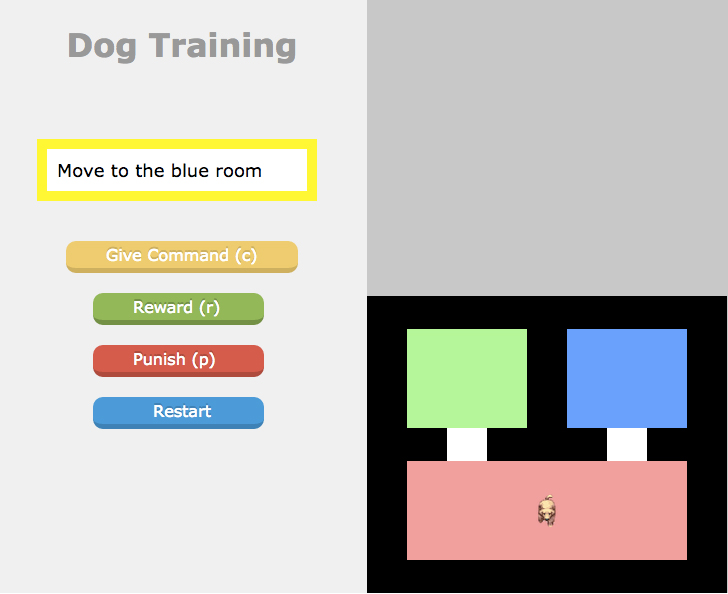
Virtual environments for training a robot dog (credit: Washington State University)
Researchers at Washington State University are using ideas from animal training to help non-expert users teach robots how to do desired tasks.
As robots become more pervasive in society, humans will want them to do chores like cleaning house or cooking. But to get a robot started on a task, people who aren’t computer programmers will have to give it instructions. “So we needed to provide a way for everyone to train robots, without programming,” said Matthew Taylor, Allred Distinguished Professor in the WSU School of Electrical Engineering and Computer Science.
User feedback improves robot performance
With Bei Peng, a doctoral student in computer science, and collaborators at Brown University and North Carolina State University, Taylor designed a computer program that lets humans without programming expertise teach a virtual robot that resembles a dog in WSU’s Intelligent Robot Learning Laboratory.
For the study, the researchers varied the speed at which their virtual dog reacted. As when somebody is teaching a new skill to a real animal, the slower movements let the trainer know that the virtual dog was unsure of how to behave, so trainers could provide clearer guidance to help the robot learn better.
The researchers have begun working with physical robots as well as virtual ones. They also hope to eventually also use the program to help people learn to be more effective animal trainers.
The researchers recently presented their work at the international Autonomous Agents and Multiagent Systems conference, a scientific gathering for agents and robotics research. Funding for the project came from a National Science Foundation grant.
Bei Peng/WSU | Dog Training — AAMAS 2016
Abstract of A Need for Speed: Adapting Agent Action Speed to Improve Task Learning from Non-Expert Humans
As robots become pervasive in human environments, it is important to enable users to effectively convey new skills without programming. Most existing work on Interactive Reinforcement Learning focuses on interpreting and incorporating non-expert human feedback to speed up learning; we aim to design a better representation of the learning agent that is able to elicit more natural and effective communication between the human trainer and the learner, while treating human feedback as discrete communication that depends probabilistically on the trainer’s target policy. This work entails a user study where participants train a virtual agent to accomplish tasks by giving reward and/or punishment in a variety of simulated environments. We present results from 60 participants to show how a learner can ground natural language commands and adapt its action execution speed to learn more efficiently from human trainers. The agent’s action execution speed can be successfully modulated to encourage more explicit feedback from a human trainer in areas of the state space where there is high uncertainty. Our results show that our novel adaptive speed agent dominates different fixed speed agents on several measures of performance. Additionally, we investigate the impact of instructions on user performance and user preference in training conditions.